Martin Dudek
MModular
•Created by Martin Dudek on 10/18/2024 in #community-showcase
Mojo added to SpeedTests repo on github
it seems the github repo owner is very open for further PRs even so maybe good not to bombard him too frequently with PRs 😉
16 replies
MModular
•Created by Martin Dudek on 10/18/2024 in #community-showcase
Mojo added to SpeedTests repo on github
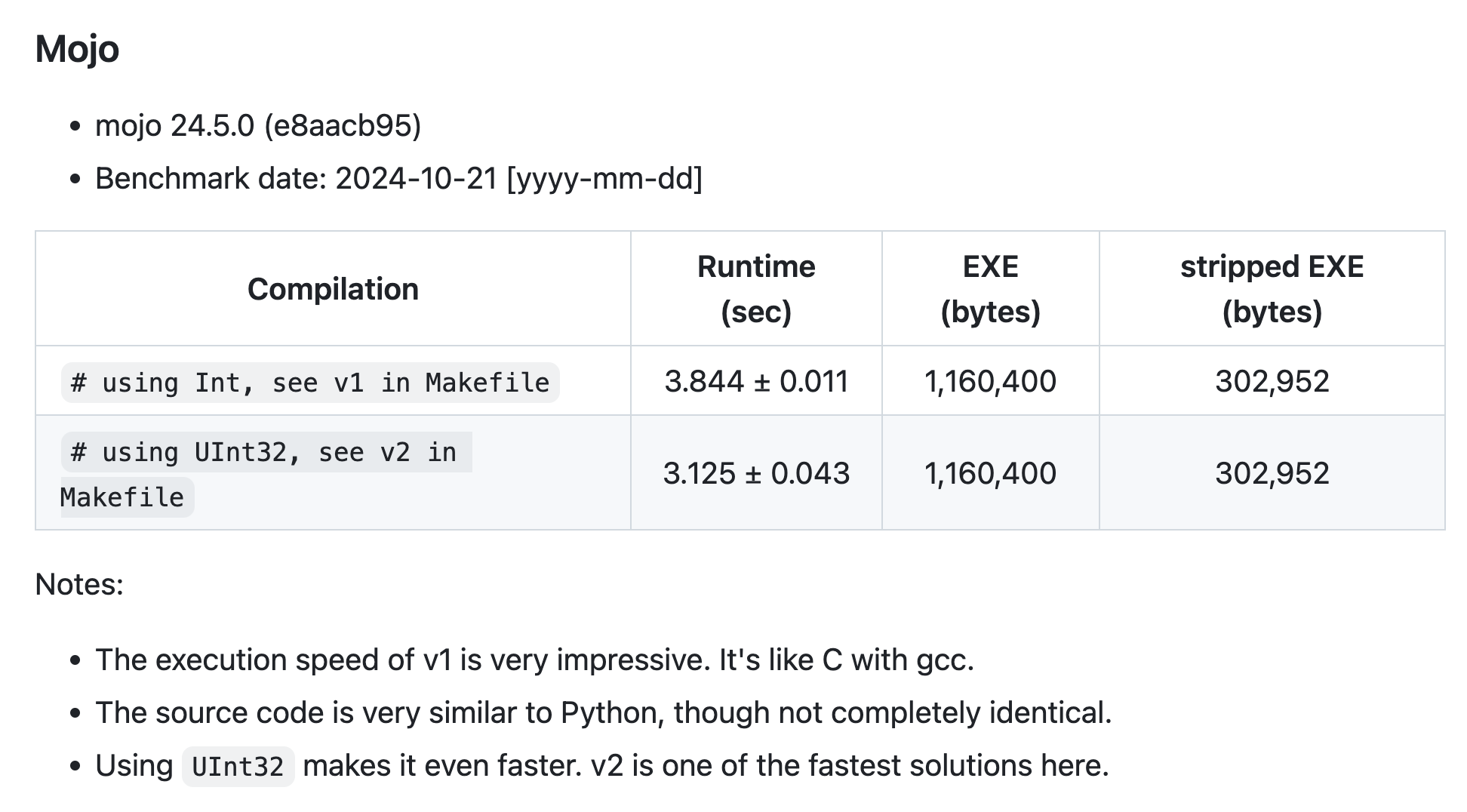
16 replies
MModular
•Created by Martin Dudek on 10/18/2024 in #community-showcase
Mojo added to SpeedTests repo on github
Some insightful comments by @Owen Hilyard and @Martin Vuyk on various ways how this simple task can be implemented appeared on github https://github.com/jabbalaci/SpeedTests/pull/63 :mojo:
16 replies
MModular
•Created by Martin Dudek on 10/18/2024 in #community-showcase
Mojo added to SpeedTests repo on github
There are only five numbers printed by the program, so this change likely won't have a noticeable impact on performance. When I run the program locally, it seems that the current nightly version is actually performing a bit slower for some reason. Let's wait for the next stable version to see if there’s a noticeable performance improvement. If there is, I'll request the GitHub repository owner to rerun the benchmark with the latest version.
16 replies
MModular
•Created by Martin Dudek on 10/18/2024 in #questions
List of references in Mojo
this looks great, thanks a lot
8 replies
MModular
•Created by Martin Dudek on 10/18/2024 in #questions
List of references in Mojo
It would be great if someone could post how to implement this example with References (and/or Arc) - never used them so not sure how to do it
8 replies
MModular
•Created by Martin Dudek on 10/18/2024 in #questions
List of references in Mojo
This will copy the lists as far as i see:
8 replies
MModular
•Created by Sören on 7/29/2024 in #questions
Any way to work around that capturing closures cannot be materialized as runtime values?
thx
8 replies
MModular
•Created by Martin Dudek on 10/11/2024 in #community-showcase
Mojo dictionary benchmarks
No, I don't have anything to say about it — the folks actively engaged in the discussion here would be the best ones to comment on dictionary performance and what to expect in the future ...
69 replies
MModular
•Created by Sören on 7/29/2024 in #questions
Any way to work around that capturing closures cannot be materialized as runtime values?
Did you find a workaround for that? Facing the same limitation here. Thx
8 replies
MModular
•Created by Martin Dudek on 10/11/2024 in #community-showcase
Mojo dictionary benchmarks
wonderful to hear that all this knowledge is flowing into stdlib dict, thanks to everyone involved in this 🙏 :mojo:
69 replies
MModular
•Created by Martin Dudek on 10/11/2024 in #community-showcase
Mojo dictionary benchmarks
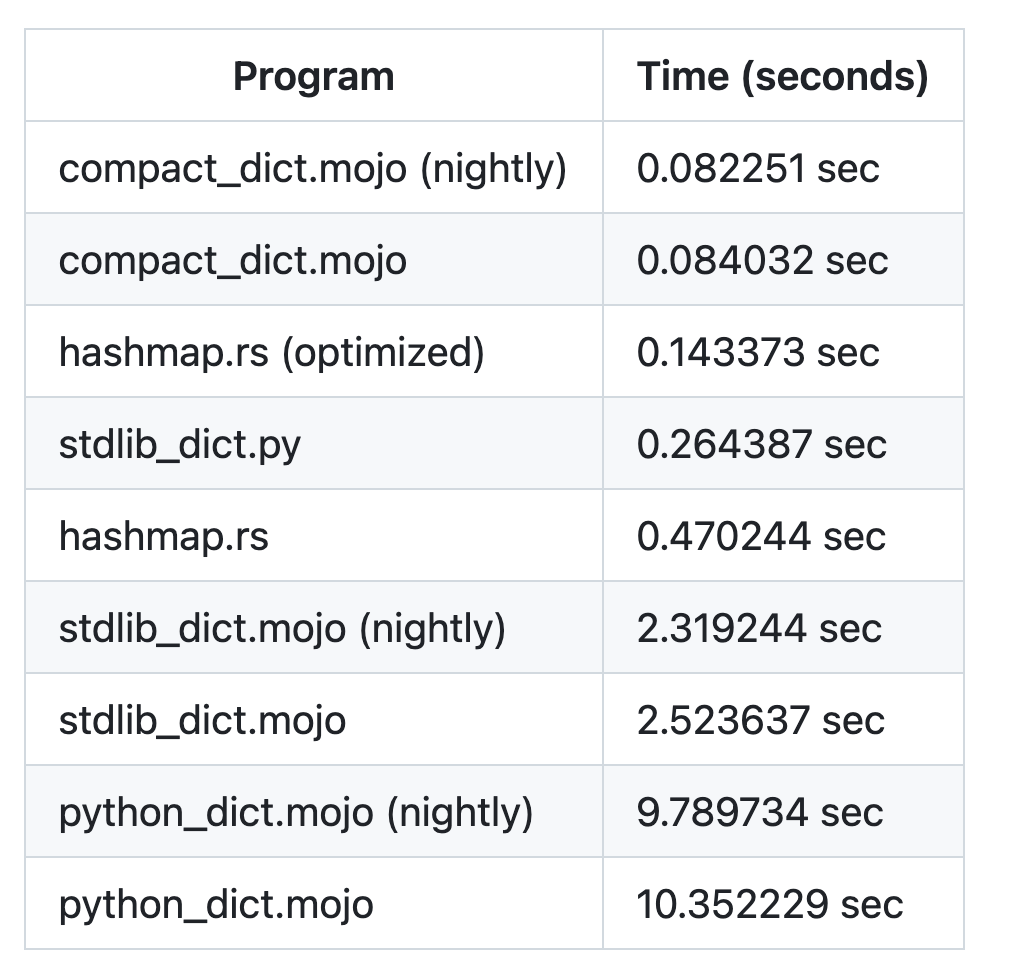
69 replies
MModular
•Created by Martin Dudek on 10/11/2024 in #community-showcase
Mojo dictionary benchmarks
just played around with creating the String keys outside the benchmark and as @Maxim already assumed, it changes the picture - i will update the repo later today
69 replies
MModular
•Created by Martin Dudek on 10/11/2024 in #community-showcase
Mojo dictionary benchmarks
very happy for all contributions as i am not an expert on these matters at all , just saw a need to look into dictionary performance.
69 replies
MModular
•Created by Martin Dudek on 10/11/2024 in #community-showcase
Mojo dictionary benchmarks
@Owen Hilyard very welcome to make a pull request for that or i do it if you prefer
69 replies
MModular
•Created by Martin Dudek on 10/11/2024 in #community-showcase
Mojo dictionary benchmarks
thanks @Maxim i am more than happy to accept any pull request if you have the time to improve this repo - as said i just put it quickly together as starting point.
69 replies
MModular
•Created by Martin Dudek on 9/19/2024 in #questions
parallelize and @parameter issue in Mojo 24.5
thanks @sora , i just tried the little demo i posted with the current nightly (mojo 2024.9.2305 - f7b111af) and yeah, it worked as expected
6 replies
MModular
•Created by Martin Dudek on 9/19/2024 in #questions
parallelize and @parameter issue in Mojo 24.5
thanks a lot @sora
6 replies
MModular
•Created by Martin Dudek on 9/16/2024 in #questions
to_numpy with Mojo 24.5
I ended up using @DobyDabaDu implementation which works like a charm. Thanks again for the all the feedback :mojo:
9 replies
MModular
•Created by Martin Dudek on 9/16/2024 in #questions
to_numpy with Mojo 24.5
thanks @TilliFe , @Darin Simmons and @DobyDabaDu - quite some other 24.4 -> 24.5 issues in the project i am working on so I cant check what works right now but will post here once it's done .... Thanks again 🙏
9 replies